Solutions for the reuse of microplates and pipette tips
Reuse is cost-effective and the preferred method to reduce dependence on single-use plastics
Microplate Cleaning Solutions
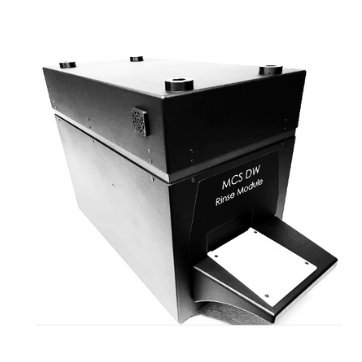
PurePLATE MCS
An integrated cleaning system for all commonly used microplates having a skirt and are 44 mm or thinner.

X-Cellerate Surface Treatment System
Grow adherant cells in microplates up to twice as fast with IonField’s proprietary plasma surface activation process
Pipette Tip Cleaning Solutions
PureTIP LHC
On-deck plasma cleaning solution for Pipette Tips and PinTools – 96, 384, 1536 channels. Compatible with virtually all brands of Liquid Handlers.
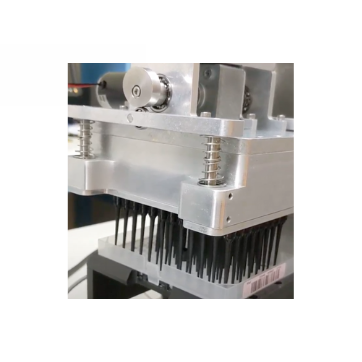
PureTIP SaS - COMING SOON
Standalone cleaning solution for racked Pipette Tips. Prototype testing focused on 1mL conductive filter tips.